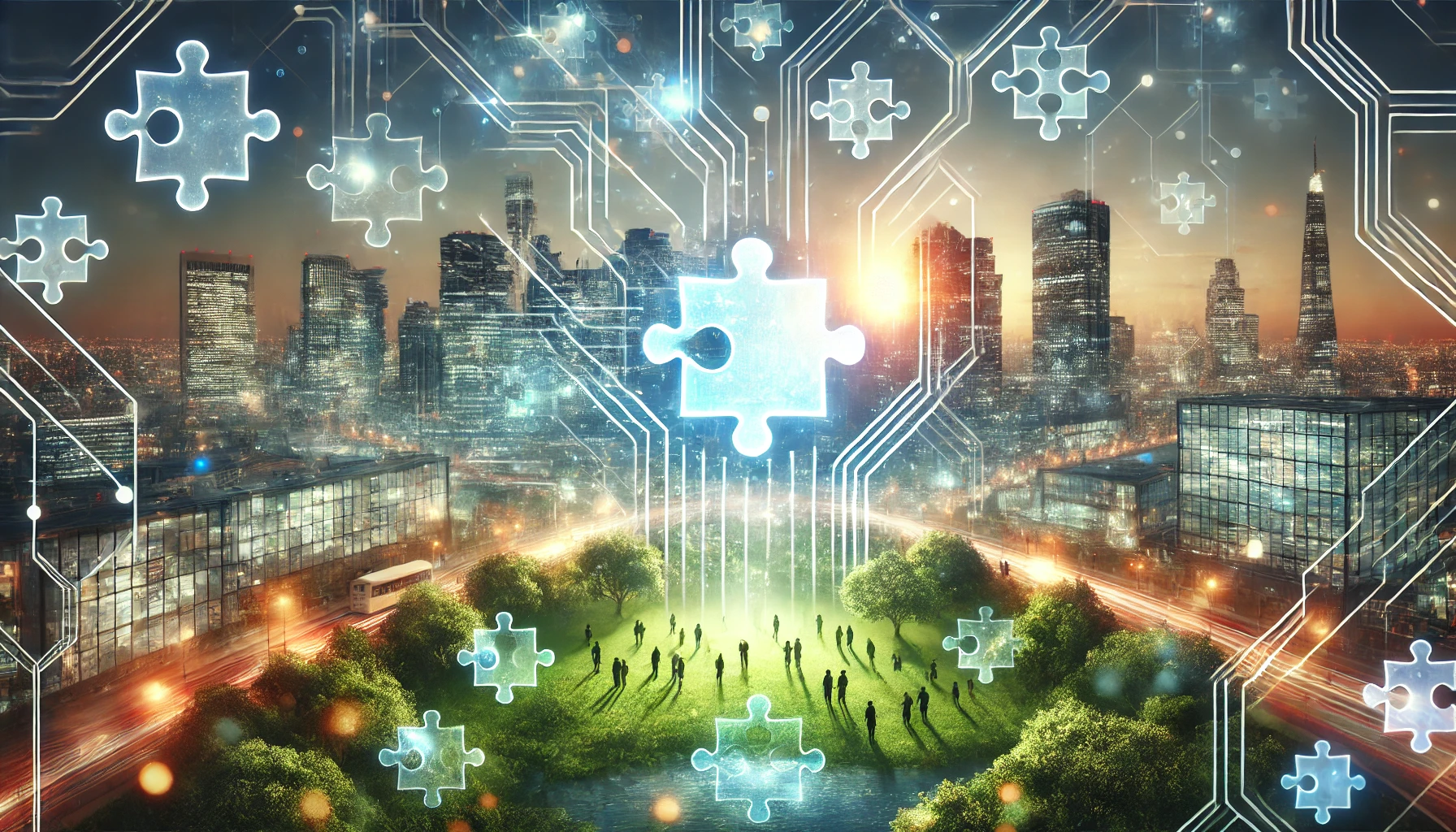
I’ve been thinking a lot about how Artificial Intelligence (“AI”) could be used to dramatically improve society – but why that doesn’t seem to be happening. I’d like to talk about why many of those efforts for good appeared to have stalled.
For the purpose of this paper, let’s call this concept of using AI to solve the biggest humanitarian efforts in the world, “AI for Good.” This isn’t an initiative that is widely discussed in the media. Most attention is focused on theoretical negatives and scare tactics around AI: dialogue about robots taking over, unstoppable technological warfare, and everyone out of jobs forever. Focus is also naturally on the things that are “cool” (like ChatGPT). To be fair, advancements like ChatGPT have been a revelation and a revolution in technology. And those developments rightfully captivate people.
But one thing we don’t talk about enough, is AI for Good. And one reason for this lack of dialogue is that it seems unfathomable that AI could solve some of humanity’s biggest problems and our collective largest pains. Think of using AI to stop human trafficking, eliminate accidental Fentynal deaths, or eradicate homelessness. To most, it is impossible to imagine what the solution to these problems would even look like. But those impossible solutions are exactly what AI can tackle and resolve. We just have to begin engaging in dialogue and working together to deploy AI against those problems.
I’ve been wanting to write down my thoughts on this concept for a while actually, because it has frustrated me for almost a decade now to watch so much time being spent on AI – but without any real progress being made in these humanitarian areas. And I’ve been reflecting on why that is; I’ve been trying to pinpoint my thoughts on why we haven’t been able to solve these problems. And to me, it comes down to a few factors.
First: AI models are created from data. Much like the human brain, models evolve by learning. Consider children. The better the teachers, education, and even early conversations that children are exposed to, the better those children can observe, repeat, internalize, and then build on those learnings. Then those children start to make their own decisions later on, based on those foundations.
AI is very, very similar. AI’s teacher is data. The better the data (accurate, complete, diverse); the more data there is (multiple sources, a lot to experiment with); and the more the models can spend with that data (practicing, and practicing again); the better the results of the model. But the inverse is true: without good data, without a large volume of it, and without the time to learn and train and iterate, AI models can’t become sophisticated enough to solve truly complex problems. Or worse, these models become mistake prone or ineffective and reinforce the narrative that the problem is impossible to solve.
Let’s talk more about these complex humanitarian problems, referenced earlier in my introduction. Let’s take human trafficking. If we are sitting in a living room having a conversation about the evils of the world, and we agree we’d like to eradicate human trafficking… the natural conversational response is, “But how?” There are thousands of examples and reasons why people have tried and failed to stem the growth of trafficking. So far, it cannot be solved (and in fact, the problem just worsens everyday). It is so daunting. It feels impossible to even identify where to start.
This idea of determining “where to start” is one that I have spent a lot of my life discussing. I’ve written about it in my blog post called the “Butterfly Principle”. The Butterfly Principle is about looking at the end goal that we want to achieve, and working backwards in a step-by-step process, to identify a starting point.
The reason why this Butterfly Principle is so important is because it’s hard for most people to start on an empty whiteboard, with just an end goal, and translate that into actionable steps. But by the end of this writing, I’m going to give you a lot of ideas that are starting points on this “empty whiteboard”. These ideas will be starting points for how to use AI for Good, and will hopefully be a starting point so people can easily take a metaphorical red pen to the words, and start editing and iterating with their own ideas.
The first words I’m writing on our imaginary whiteboard – designed to get our thoughts flowing on how to use AI for Good to eradicate human trafficking – are “Data Sharing”.
Data Sharing
Going back to our discussion on how AI trains: a good model (especially one sophisticated enough to solve this endlessly complex problem) needs a lot of good data and from many sources. Why don’t we just give AI models data, let them train happily away? If only it was that easy. Many think that the data to solve these problems doesn’t exist; it absolutely does. But the challenging part is getting at the data.
While the data exists, it does so in data silos. This means that it is owned by different groups of people, different companies (different divisions within those companies), different government agencies, different organizations – most entirely unrelated to the others. Most of these entities are unwilling to share their data with other segments of their own organization, let alone outside of their organization. Data silos have become giant fiefdoms, where it is all “mine” and not yours. And data has become people’s gold. For years now, the dialogue in society is all about how “data is the future”, and is something that is valuable, something that can be monetized, something that is a competitive advantage, and also something that can be harmful if leaked or intentionally shared with adverse parties. So generally speaking, why would anyone ever share their data?
Now, let’s imagine we approached various people and entities, and asked for their data – specifically for the purpose of eradicating human trafficking. But they still say no. Are they unwilling to share because they don’t want to solve these large problems? I’ve never found that to be the actual reason. It is more that they don’t want to share because data has been misused overtime. Their willingness to share has been exploited in previous endeavors or maybe even personal information was exposed. Protecting people’s PII (Personal Identifiable Information) is critical to the success of sharing data. In fact, the sharing of PII isn’t even needed to solve these problems. Ultimately, data owners don’t understand how their data (which is just one piece to a large puzzle) can help solve issues that are truly meaningful. The “bigger picture” is not clear.
The next words I’m writing on our imaginary whiteboard are “Seeing is Believing” and “Puzzle Pieces”.
Seeing is Believing; Puzzle Pieces
One of the only ways we’re ever going to solve for the mass sharing of data is going to be gaining trust from the people who have that data. Trust can be gained based on the old adage of “Seeing is Believing”. We have to be able to show a given person (organization) how their one, single piece of a puzzle (the data they can supply) fits into the bigger picture. We can’t just tell them this – they have to literally see what we’re talking about. They must see how they make a substantial difference.
Imagine trying to put together a puzzle on your kitchen table. You’re struggling to find any pieces that fit together. Imagine also that some of your family members have some key pieces, like corners. The only way you’ll solve the puzzle is if you can convince them to contribute their pieces. Pretend your sister is holding a corner piece, but she is in the living room, sitting on a very comfortable sofa, watching a college football game. She hasn’t seen the picture on the back of the box – she doesn’t know how beautiful the end result can be. She doesn’t know what the goal is. She doesn’t understand that you’re hoping to complete the puzzle and frame it in your kitchen, for everyone to enjoy. Without any of that background, her motivation to get off the sofa and give you her corner piece will rightfully be low.
But what if you could show her the final goal – and she could see that by giving you the corner, you’ll get unstuck, you’ll make progress, and ultimately your family will have a beautiful puzzle solved and framed in the kitchen for them all to enjoy? She can see, believe, and participate to help the “greater good”.
That’s what we would do here, in our AI for Good example. We need to educate potential data contributors on the end goal… and the incremental steps to get to that goal. Potential contributors will range from government agencies, to private companies, to public companies like social networks, to everyday people. But we must have a plan on how to go and talk to them about how their contribution fits into the bigger picture. If we have worked the problem backwards and have every step of the way mapped out, we can have those conversations; we can show them – seeing is believing.
But with that said, we have to be realistic. And for some entities, even the most persuasive discussion of the puzzle will not be enough to convince them to share their data. What next? Do we give up?
The next words I’m writing on our whiteboard are “Solving Headaches”.
Solving Headaches
Sometimes entities need a bigger carrot to participate in data sharing; what is needed is a symbiotic relationship. In biology, a symbiotic relationship is “mutualistic”, one where both parties involved benefit from the interaction. In this application: I have found that if you can find a solution for someone’s problem – a headache they have – and your provided solution is using the same data that is needed to solve the AI for Good problem – then, these entities are open to a sharing in a “symbiotic [data] relationship”.
Some people question why we should have to build something just to “bribe” entities to contribute to solving a societal issue. That’s not the right question – the only question is how to solve the societal issue. If that requires entering symbiotic relationships with various entities – that has to be fine. The sole focus needs to be promoting AI for Good, and that means determining how we make entities comfortable sharing data. We just need to focus on getting each individual piece of the puzzle. And if that means that we have to go down side paths that outwardly have nothing to do with the main goal – but have everything to do with getting that critical data – then that is time well spent. It is critical to accomplishing the goal we all want. In this example, to forever end human trafficking.
We must figure out a way to eliminate data fiefdoms and silos within companies and governments. Because these silos are so hardened, it will not be an easy task. But if we do not address this issue, we will be in a worse position, where we get stuck as a society in a situation where companies and governments just won’t share to begin with. We will only make minimal progress at best, adding “false hope” to solving the real problem. Of course, as users of technology, everyday people are sharing their data all of the time. Some of this is willingly, some unwillingly and unknowingly. While users need more control over their data, this is a different issue and shouldnt be conflated with the sharing needed by companies and governments (although sometimes it is the sharing of user data, hence the previous comments about protecting PII and everyone’s right to privacy).
I’ve seen firsthand what happens when you can show people a vision, and explain specifically why their data helps you get to your end result in a micro-step. And when you get that data and deliver on the promised micro-result, they are likely to keep contributing. This cycle feeds on itself. More and more people contribute; more and more micro-problems get solved; progress is made.
The bottom line is that we have to think radically differently if we are ever going to see a world where AI for Good is deployed to solve global humanitarian problems, and is positively altering our societal fabric forever. We need to change the conversation from “using AI means that a robots takeover is inevitable” to “responsibility developing AI means that families would no longer have to worry about their children being taken into human trafficking”. We can solve these problems. It is not impossible. We just need to be willing to think about approaching the problem in a way that might feel unnatural: the first step is to get good data, the lifeblood of training AI for Good.